{ "cells": [ { "cell_type": "markdown", "metadata": {}, "source": [ "" ] }, { "cell_type": "markdown", "metadata": {}, "source": [ "# Course Notes for Learning Intelligent Systems" ] }, { "cell_type": "markdown", "metadata": {}, "source": [ "Department of Telematic Engineering Systems, Universidad Politécnica de Madrid, © Carlos A. Iglesias" ] }, { "cell_type": "markdown", "metadata": {}, "source": [ "## [Introduction to Machine Learning II](3_0_0_Intro_ML_2.ipynb)" ] }, { "cell_type": "markdown", "metadata": {}, "source": [ "# Table of Contents\n", "\n", "* [The Titanic dataset](#The-Titanic-dataset)\n", "* [Reading Data](#Reading-Data)\n", "* [Reading Data from a File](#Reading-Data-from-a-File)" ] }, { "cell_type": "markdown", "metadata": {}, "source": [ "# The Titanic dataset" ] }, { "cell_type": "markdown", "metadata": {}, "source": [ "In this session, we will work with the Titanic dataset. This dataset is provided by [Kaggle](http://www.kaggle.com). Kaggle is a crowdsourcing platform that organizes competitions where researchers and companies post their data and users compete to obtain the best models.\n", "\n", "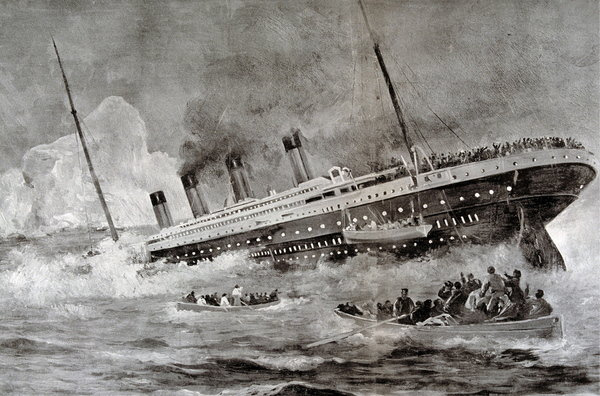\n", "\n", "\n", "The main objective is to predict which passengers survived the sinking of the Titanic.\n", "\n", "The data is available [here](https://www.kaggle.com/c/titanic/data). There are two files, one for training ([train.csv](files/data-titanic/train.csv)) and another file for testing [test.csv](files/data-titanic/test.csv). A local copy has been included in this notebook under the folder *data-titanic*.\n", "\n", "\n", "Here follows a description of the variables.\n", "\n", "| Variable | Description | Values |\n", "|------------|---------------------------------|-----------------|\n", "| survival | Survival |(0 = No; 1 = Yes)|\n", "| Pclass | Name | |\n", "| Sex | Sex | male, female |\n", "| Age | Age | |\n", "| SibSp |Number of Siblings/Spouses Aboard| |\n", "| Parch |Number of Parents/Children Aboard| |\n", "| Ticket | Ticket Number | |\n", "| Fare | Passenger Fare | |\n", "| Cabin | Cabin | |\n", "| Embarked | Port of Embarkation | (C = Cherbourg; Q = Queenstown; S = Southampton)|\n", "\n", "\n", "The definitions used for SibSp and Parch are:\n", "* *Sibling*: Brother, Sister, Stepbrother, or Stepsister of Passenger Aboard Titanic\n", "* *Spouse*: Husband or Wife of Passenger Aboard Titanic (Mistresses and Fiances Ignored)\n", "* *Parent*: Mother or Father of Passenger Aboard Titanic\n", "* *Child*: Son, Daughter, Stepson, or Stepdaughter of Passenger Aboard Titanic" ] }, { "cell_type": "markdown", "metadata": {}, "source": [ "# Reading Data" ] }, { "cell_type": "markdown", "metadata": {}, "source": [ "In the previous dataset we load a bundle dataset in scikit-learn. In this notebook we are going to learn how to read from a file or a url using the Pandas library." ] }, { "cell_type": "markdown", "metadata": {}, "source": [ "## Reading Data from a File" ] }, { "cell_type": "code", "execution_count": null, "metadata": {}, "outputs": [], "source": [ "import numpy as np\n", "import pandas as pd\n", "from pandas import Series, DataFrame\n", "\n", "df = pd.read_csv('data-titanic/train.csv')\n", "df" ] }, { "cell_type": "code", "execution_count": null, "metadata": {}, "outputs": [], "source": [ "# we can get the number of samples and features\n", "df.shape" ] }, { "cell_type": "code", "execution_count": null, "metadata": {}, "outputs": [], "source": [ "#I can read only a number of rows and tell where the header is, among other options.\n", "df = df = pd.read_csv('data-titanic/train.csv', header=0, nrows=5)\n", "df" ] }, { "cell_type": "markdown", "metadata": {}, "source": [ "Pandas provides methods for reading other formats, such as Excel (*read_excel()*), JSON (*read_json()*), or HTML (*read_html()*), look at the [documentation](http://pandas.pydata.org/pandas-docs/stable/api.html#input-output) for more details." ] }, { "cell_type": "markdown", "metadata": {}, "source": [ "## Reading data from a URL" ] }, { "cell_type": "code", "execution_count": null, "metadata": {}, "outputs": [], "source": [ "import pandas as pd\n", "#We get a URL with raw content (not HTML one)\n", "url = \"https://raw.githubusercontent.com/gsi-upm/sitc/master/ml2/data-titanic/train.csv\"\n", "df = pd.read_csv(url)\n", "df" ] }, { "cell_type": "markdown", "metadata": {}, "source": [ "An alternative option is reading the file with the library *requests* and then use *pandas*." ] }, { "cell_type": "code", "execution_count": null, "metadata": {}, "outputs": [], "source": [ "# First we open the file\n", "import pandas as pd\n", "import io\n", "import requests\n", "url = \"https://raw.githubusercontent.com/gsi-upm/sitc/master/ml2/data-titanic/train.csv\"\n", "s = requests.get(url, stream=True).content\n", "#Print the first 320 characters for understanding how it works\n", "s[:320]" ] }, { "cell_type": "code", "execution_count": null, "metadata": {}, "outputs": [], "source": [ "df = pd.read_csv(io.StringIO(s.decode('utf-8')))\n", "df" ] }, { "cell_type": "markdown", "metadata": {}, "source": [ "## References" ] }, { "cell_type": "markdown", "metadata": {}, "source": [ "* [Pandas API input-output](http://pandas.pydata.org/pandas-docs/stable/api.html#input-output)\n", "* [Pandas API - pandas.read_csv](http://pandas.pydata.org/pandas-docs/stable/generated/pandas.read_csv.html)\n", "* [DataFrame](http://pandas.pydata.org/pandas-docs/stable/dsintro.html)\n", "* [An introduction to NumPy and Scipy](https://sites.engineering.ucsb.edu/~shell/che210d/numpy.pdf)\n" ] }, { "cell_type": "markdown", "metadata": {}, "source": [ "## Licence" ] }, { "cell_type": "markdown", "metadata": {}, "source": [ "The notebook is freely licensed under under the [Creative Commons Attribution Share-Alike license](https://creativecommons.org/licenses/by/2.0/). \n", "\n", "© Carlos A. Iglesias, Universidad Politécnica de Madrid." ] } ], "metadata": { "kernelspec": { "display_name": "Python 3", "language": "python", "name": "python3" }, "language_info": { "codemirror_mode": { "name": "ipython", "version": 3 }, "file_extension": ".py", "mimetype": "text/x-python", "name": "python", "nbconvert_exporter": "python", "pygments_lexer": "ipython3", "version": "3.7.1" }, "latex_envs": { "LaTeX_envs_menu_present": true, "autocomplete": true, "bibliofile": "biblio.bib", "cite_by": "apalike", "current_citInitial": 1, "eqLabelWithNumbers": true, "eqNumInitial": 1, "hotkeys": { "equation": "Ctrl-E", "itemize": "Ctrl-I" }, "labels_anchors": false, "latex_user_defs": false, "report_style_numbering": false, "user_envs_cfg": false } }, "nbformat": 4, "nbformat_minor": 1 }